Abstract Detail
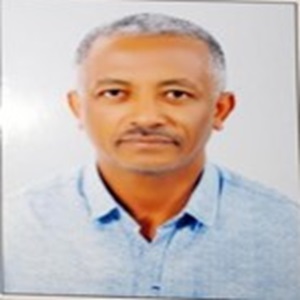
Abebe Alemu
Adama Science and Technology University, Ethiopia
Abstract
The ongoing severity of breast cancer and its death toll rate has continued to be a global concern. To this effect, the existing diagnosis and treatment are not efficient enough to successfully detect both the stage and type of breast cancer tumours at its early stage. Recent studies reported that 25.84% of all cancer- related deaths are from breast cancer. It also mentioned number of new breast cancer cases amounted to 29.46% and 31.85% of the total new cancer cases in Africa and Ethiopia, respectively. Researchers in the area agreed that among the critical areas to address in any efficient early detection model of breast cancer is to improve values associated with the false positive and false negative. Artificial intelligence, machine learning, deep learning, and computational intelligence are techniques that most researchers utilize to address the problem and continue the study to present different solutions. Most breast cancer diagnoses are in an advanced stage (95% CI; 68.46-76.65%), highlighting the urgent need for early detection. Furthermore, the lack of radiologists contributes to delays in the annual reading and classification of BI-RAD. It is important to note that most women diagnosed with breast cancer receive negative or non-invasive results. A retrospective research project focuses on screening breast mammography images to identify the level of BI-RAD. We develop a hybrid computational intelligence framework and implement an OpenCV for image classification, hybridizing genetic algorithms with K-Means++ to optimize image clustering. The model accurately screens BI-RAD levels and provides results for patients based on the recommendations of senior radiologists. This paper implements a comprehensive hybrid intelligent model using computational intelligence and machine learning for the early detection and classification of breast tumours. In effect, addresses improving both the True Positive and True Negative Results. Showcase: • Hybrid model of GA-KNN-SVM • Classification Process of the Breast Image • The result of the clustering of the image as BI-RAD 0,1,2,3 and the remaining considered cancerous and need critical diagnosis. • Performance measure result: Accuracy, Sensitivity, Specificity, Recall, F1-Score, NPVBiography
I am a PhD candidate in computer science and engineering specializing Computational intelligence. I have MSc in Computational Science& MSc Computer Science, and BSc Computer Science and BEd Mathematics. I am working as a researcher, consultant, and lecturer within the field of ICT; consulting in software development, system analysis, and design. I have different short-term certifications and published research papers, like Computational optimization usingepidemiological models. My PhD research work is computational intelligence in the domain area of health, specifically the detection and prediction of breast cancer.